21 Oct Cropland requirement in DELTA Model® scenarios
How much land will we need to feed the global population in the future? And what should be grown on it? The SNi team are currently working on modelling the required cropland under different future food production scenarios. This will feature in the upcoming DELTA Model® version 2.0 and an accompanying scientific publication.
Agricultural crops supply the majority of the food and nutrients consumed by the global population. As the population grows, so too does the need and demand for these foods. Meeting this increased demand will require either using more land for growing crops, efficiency gains, and/or changes to production patterns. Forecasting what this will mean for the food system is challenging.
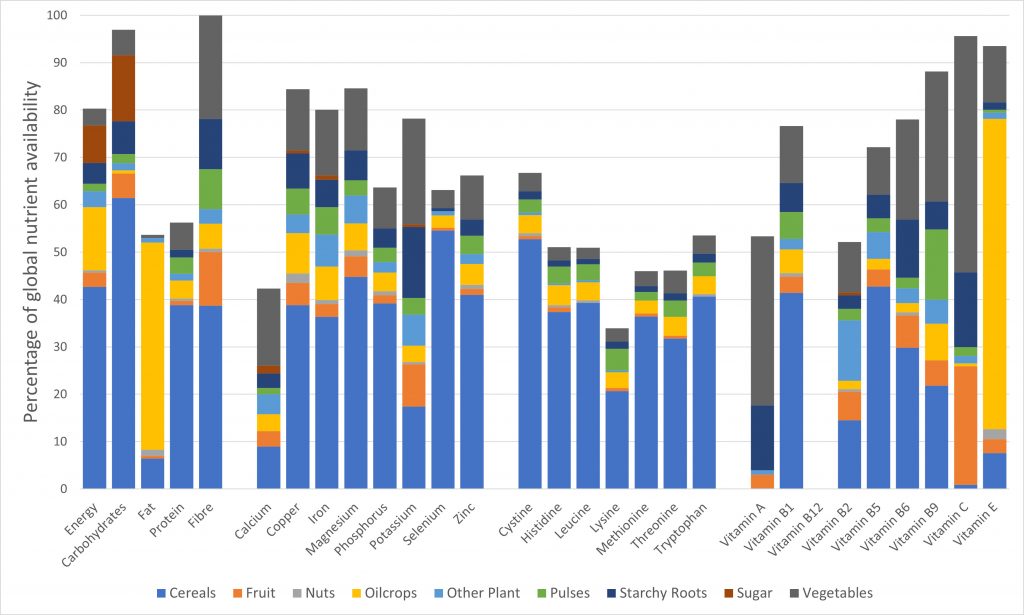
Contribution of plant foods to global nutrient availability, as calculated by the DELTA Model®
The current version of the DELTA Model® allows users to explore how future global food system scenarios would meet the nutritional needs of the global population. However, it does not consider the natural resources needed to support these scenarios, such as water and land.
As a first step towards this, the SNi team have developed a cropland model to be added to the DELTA Model®. This model does not include grazing or rangeland, but solely the agricultural land growing human-edible crops. Here, we report some of the early results of this modelling.
Wheat as an example
If we look at the production of wheat in all countries that grow it, we can analyse which countries have the highest and lowest production, area harvested, and yield (tonnes produced per hectare harvested). We can then break down global wheat production by performance.
Global total | Highest yielding 10% of production | Highest yielding 50% of production | Poorest yielding 50% of production | Poorest yielding 10% of production | |
Production (million tonnes) | 734 | 73.4 | 367 | 367 | 73.4 |
Land area harvested (million hectares) | 214.3 | 10.5 | 75.2 | 139.1 | 41.0 |
Yield (tonnes/hectare) | 3.43 | 7.02 | 4.88 | 2.64 | 1.79 |
The highest yielding 50% of wheat production in 2018 used only 75.2 million hectares, 35% of the total area of wheat harvested globally. Even better, the highest yielding 10% of production used less than 5% of the total area, compared to 19% for the poorest yielding 10%.
We can use these values to predict the land area required if wheat production were increased in the future, or what would happen to production if some of the poorer yielding countries were able to improve their performance.
For example, if wheat production needed to increase by 30% to meet the needs of the future population, how much land would be required?
If we just assume the additional production were at the global average yield, then we would need an extra 30% compared to the current land producing wheat, which is about 65 million extra hectares.
But, if the additional production happened in countries that can achieve high yields, the extra land required could be less than 45 million hectares. Conversely, if the extra production were forced onto marginal, poor yielding land, the area required could be over 85 million hectares.
But what about future changes to existing yields?
If the poorest yielding half of wheat production were to improve to match the yields of the best performing half, the extra 30% of required production could comfortably be met without increasing the land area required.
However, is such a performance increase possible in reality?
Uncertainty in future crop yields stems from many factors: technology and management practices could improve; the climate could change our ability to grow wheat in different parts of the world; local conflicts may emerge and disrupt farming; pollinator-dependent crops may be at risk of reduced fertility, and so on. It is essential to include this uncertainty when forecasting future food system scenarios, for example by considering the results under both high and low yield conditions.
Analysing individual crops and crop groups
The approach applied to wheat can be used on any crop or group of crops. This lets us consider the potential to improve crop yields in the future.
The simplest theoretical way to see the crops with greatest potential to improve yields is to compare the yield of the best performing half of production with the yield of the worst performing half. The greater the difference between these numbers, the greater the potential for improvement.
Cashew nuts are at the top of the list for this improvement potential. The average yield of the top half of production was about 9 tonnes per hectare, compared to 0.5 tonnes per hectare for the bottom half. Cashews were followed by papayas and pistachios for improvement potential.
This does not mean that improving cashew yields is the best way to raise cropland efficiency. Cashew production is small compared to crops like wheat. To make the biggest difference to cropland use, it is the cereals that should be targeted. Improving poor maize yields to the performance of the best half of production would result in a 72% increase in maize from the same land area. Improving wheat (42% increase) and rice (38% increase) would also make a large difference to total food production.
Example scenarios
Incorporating the cropland modelling into the DELTA Model® lets us look at specific future scenarios. In the figure below, 2018 cropland area is compared with the forecast 2030 requirement (if food production increases at the same rate as the population). Assuming existing yields remain the same, this results in an increase in land requirement, regardless of whether the new production is at high yields or low yields (indicated by the error bars).
However, if 30% of the poorer performing production was improved as described above, the increased production could be achieved on about the same area of land as was used in 2018.
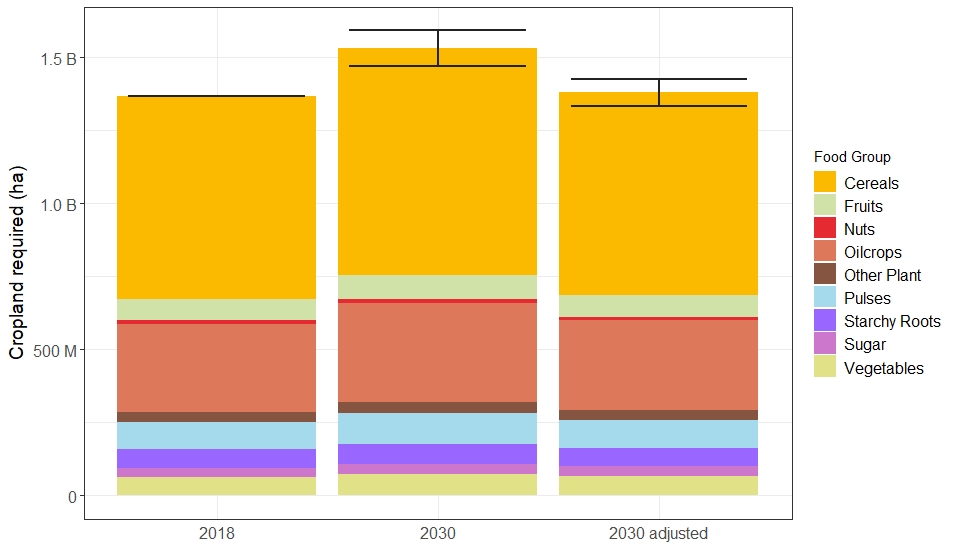
Comparing cropland requirement in 2018 with two simulated scenarios. The 2030 scenario shows predicted cropland requirement if all crop production were to increase at the same rate as the population. The 2030 adjusted scenario is identical, but 30% of poor performing production has been improved to the level of the best yielding half of production. Error bars indicate the upper and lower bounds of the prediction.
It’s important to realise that this approach to crop yields is far from perfect. The best yielding countries often have climatic, geographical, and technological advantages over the poorer yielding countries. Some of these barriers will be impossible to overcome, meaning that improving the poorer yields will not be possible. However, the approach given here is useful for identifying the crops with wide variation in their yields and for estimating future cropland requirement.
Nutritional factors should also be considered when thinking about crops to focus on improving in the future. While cereals are a good source of energy and occupy a lot of land, globally it is not energy that is deficient in the human diet, but micronutrients. Therefore, crops that are dense sources of micronutrients, such as vegetables or pulses, may be better targets for cropland use.
We also need to think about the use of crops once harvested, as not all crop biomass is directed to food. For example, over a quarter of sugar production goes towards non-food uses, such as biofuel production, while about a third of cereal production goes to animal feed. Land use selection encompasses more than just nutrition.
Finally, if cropland area is to increase, where will this land come from? There were 1.4 billion hectares of cropland in 2018, up 20% since 2000. Land conversion from forest has well-discussed disadvantages, so often grassland is proposed as a source of additional area for crop production. Research has estimated that around 685 million hectares of grassland could be converted to crops, but there are many disadvantages to this, from reducing available grazing land to impacts on biodiversity.
Assessing what crops should be grown where, and under what practices, bridges nutritional science and agricultural science. Inclusion of cropland area in DELTA Model® scenarios will give users another perspective on what might be possible, practical, and optimal in the future food system.
Photo by Henry Be on Unsplash